가장 기본적인 clustering algorithm, unsupervised.
k는 cluster의 개수를 나타낸다.(hyperparameter)
최적의 k개의 centroids를 찾는 것이 알고리즘의 목표
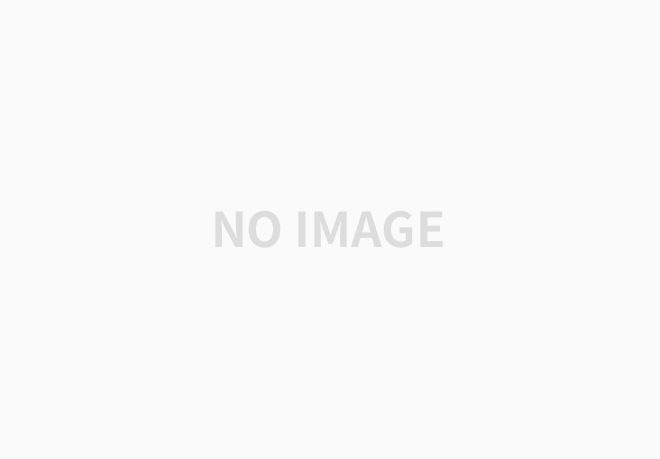
알고리즘:
Assignment-step과 Update-step으로 나뉘어져있다.
각 data마다 가장 가까운 centroid을 갖는 cluster로 assign
위의 loop를 다 돌면 centroid를 해당 cluster내의 point average를 통해 update
위 2과정을 반복 후 converge하면 알고리즘 종료
특징:
Optimum으로 convergence가 보장되어 있지 않다.
L2 distance가 아닌 distance는 더 converge가 보장 안된다.
->data의 scatter가 Sphere모양이 아닌 경우에는 clustering이 잘 되지 않는다.
->이 때는 DBSCAN을 사용하자.(DBSCAN은 다음에 알아보자.)
NP-hard for any k >= 2
복잡도가 O(n^(dk+1)), where n:데이터 개수, d:데이터 차원, k:cluster개수
->따라서 변형인 Lloyd's algorithm을 대개 사용한다. (이는 다음에 알아보자.)
metric:
clustering이 얼마나 잘됐나 평가하는 데에 있어
각 클러스터 내의 data가 해당 centroid까지의 distance,
그리고 이를 전체 data에 대해 평균내린 값을 metric으로 잡아 사용(낮을 수록 좋은)
이러한 metric을 average diameter라 한다.
최적의 k찾기:
average diameter가 k가 클수록 낮아지는 경향이 있다. 이 때 낮아지는 "폭이" 줄어들 때의 k를 최적의 k로 택한다.
참고자료:
en.wikipedia.org/wiki/K-means_clustering
k-means clustering - Wikipedia
From Wikipedia, the free encyclopedia Jump to navigation Jump to search Vector quantization algorithm minimizing the sum of squared deviations k-means clustering is a method of vector quantization, originally from signal processing, that aims to partition
en.wikipedia.org
www.secmem.org/blog/2019/05/17/clustering/
클러스터링(군집화) 개요
클러스터링(군집화) 클러스터링(군집화)은 개체들이 주어졌을 때, 개체들을 몇 개의 클러스터(부분 그룹)으로 나누는 과정을 의미합니다. 이렇게 개체들을 그룹으로 나누는 과정을 통해서, 클러
www.secmem.org
'ML' 카테고리의 다른 글
[MachineLearning] Batch Normalization (0) | 2020.11.05 |
---|---|
[Numpy]ndarray가 (built-in)list보다 빠른 이유 (0) | 2020.11.02 |
[Numpy]각 row에서 k개의 the largest values 뽑기 (0) | 2020.10.25 |
[Numpy]numpy.ndarray에서 각 row마다 특정 column의 원소를 가져오고 싶을 때 (0) | 2020.10.25 |
[Numpy]numpy.ndarray 각 원소에 dictionary map할 때 (0) | 2020.10.25 |